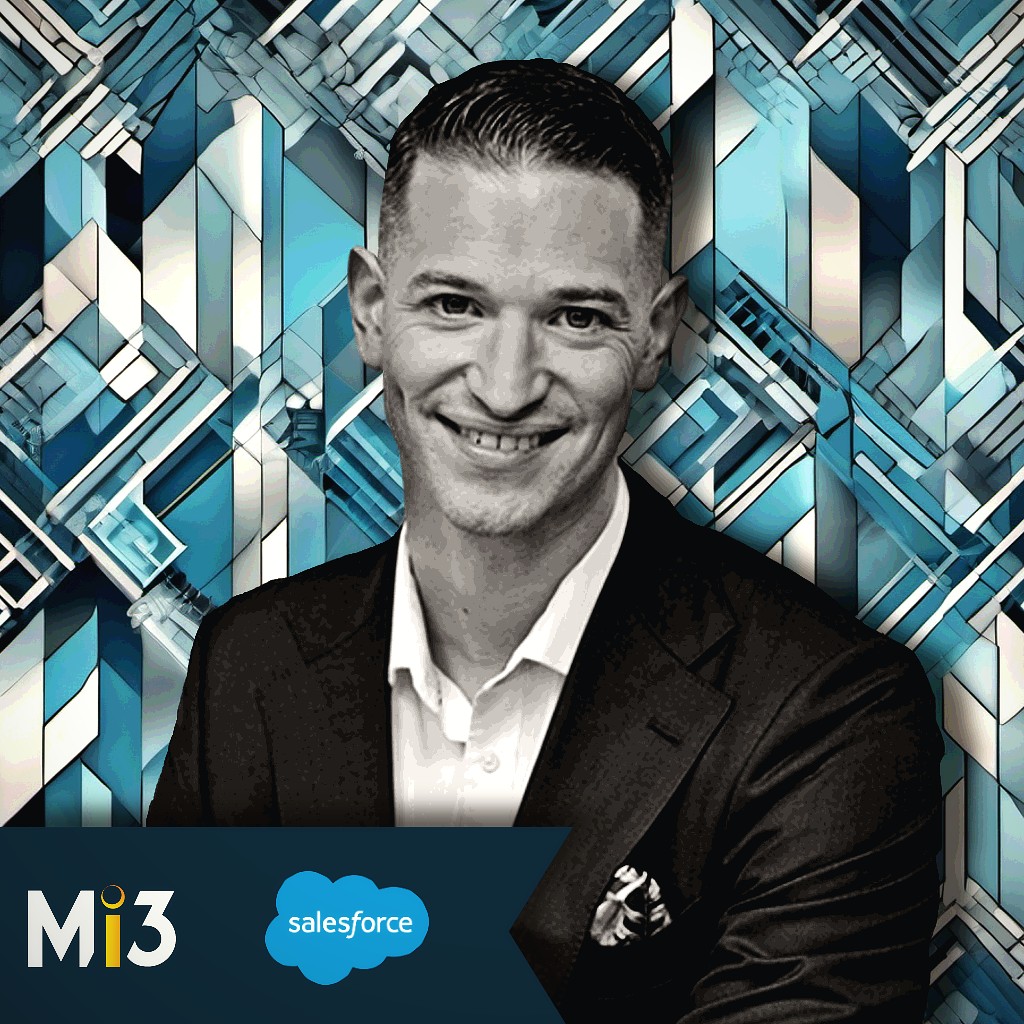
Editors' Note: Many Fast News images are stylised illustrations generated by Dall-E. Photorealism is not intended. View as early and evolving AI art!
AI's power grows,
But data's the key to thrive,
Quality must show.
Partner Op-Ed: If you think your data is ready for AI, you’re probably wrong
By Leandro Perez, Senior Vice President and CMO APAC, Salesforce
By now, you’ve probably experienced the genius of generative AI. The first time I saw it really working at its full potential, it seemed like a kind of magic.
But the truth is that to truly tap into that magic – to really make AI work for your business to deliver results – you need to combine the power of artificial intelligence with one of the greatest resources your company has: your customer data.
Without real-world data about your customers, generative AI may end up falling back on hallucinations and guesswork. And even if it doesn’t make mistakes, generic outputs from a generative AI tool that doesn’t have access to customer data can’t hope to compete with the personalised, bespoke results from one that has access to specific customer data.
It’s understandable that businesses have been accelerating their efforts to implement AI solutions as quickly as possible. But using generative AI without trusted, accurate customer data could be risky, even dangerous.
Let's say a retailer uses generative AI to craft personalised text messages or emails informing customers of order delivery status, or information about new products. If those messages aren’t crafted based on accurate data, there’s a real potential for customer communication to misfire, potentially leading to lost trust and loyalty.
The illusion of ‘good’ data
So we’ve established the importance of using customer data. But not all customer data is created equally – your company’s AI strategy is only as good as its data strategy. Just having a mountain of customer data isn’t going to be enough to leverage AI to the fullest extent.
This is where you might require a change of leadership style – as part of your data strategy you’ll need to instil a data-centric culture across your entire organisation to ensure your customer information remains of the highest quality.
So what does good data look like?
Disconnected data sets
I don’t think I could count the number of times I’ve encountered businesses that think they have ‘good’ customer relationship management, only for me to discover that their data is spread across six different systems, including paper records or the memory of a long-serving sales rep.
Let’s just get it out of the way early – if your teams have to open one system to see customer orders, another to create a marketing EDM, and a third to allow AI to craft the message, your data is disconnected.
If you don’t have one already, now is the time to invest in a data platform that provides you with a unified view of all your customer information in one place. A platform that provides a common data model onto which all your customer information can be mapped. Trying to leverage AI without one is going to lead to mistakes, omissions, and, at worst, possible regulatory trouble.
Quality vs. quantity
AI isn’t fussy when it comes to data – it’ll take anything you can feed it. But for data to be useful it needs to be accurate, accessible, clean and complete.
And I’m here to tell you – not everyone’s data meets those standards.
If you don’t have total control over your data, serious issues of bias, prejudice, or factually incorrect hallucinations can sneak into the outputs produced by your model. Biases and mistakes in your training data can have serious real-world ramifications if they result in offensive or incorrect messages being sent out in your business’s name.
The most advanced platforms, like Salesforce Einstein 1, have safeguards in place that can automatically detect and flag toxic language, and you should always have a human in the loop to review your AI model’s output.
Bottom line – bad data in, bad results out.
Ethical considerations
Do you know where your data comes from, really? Or where it goes? What LLM are you using, and what’s it doing with your data when it receives it?
These are all considerations you need to make when choosing how your data is handled by artificial intelligence, and how you make use of AI outputs.
And there are economic drivers at play, too. A lack of embedded capabilities for things like ethical data management can burden marketers with 8–12% productivity loss. That adds up. As this report says, without the right system capabilities and clear guidelines, marketing teams can find themselves ill-equipped to handle a whole new set of responsibilities like customer data compliance and reporting.
Compliance with data regulations
A good data strategy should include a complete understanding of all the relevant laws and regulations that govern data use in your industry. AI is a little like the Wild West at the moment, but you can get ahead of any potential legislation with a data strategy that includes guidance on how to respond to changes to the law, and a data platform that is robust enough to manage them.
Be careful, too, when letting untested tech access and learn from your data. New kids on the block are making bold promises, but with changes to the Australian Privacy Act, it’s best to stick with tried and tested tools.
Good AI is built on great data
This is such an important point that I want to repeat it again: without a robust and comprehensive data strategy, any business’s AI strategy is going to falter, if not fail completely.
And as well as a strategy, you’re going to need a data platform that will help you meet your business objectives – a platform that allows you to unify your disparate data sources, and by extension your entire company.
Before you jump in and start using the latest shiny generative tools, put in the time now to really, truly understand and define how your data is collected, categorised, and stored. Laying that path now will form the basis of a long and productive road ahead with AI.